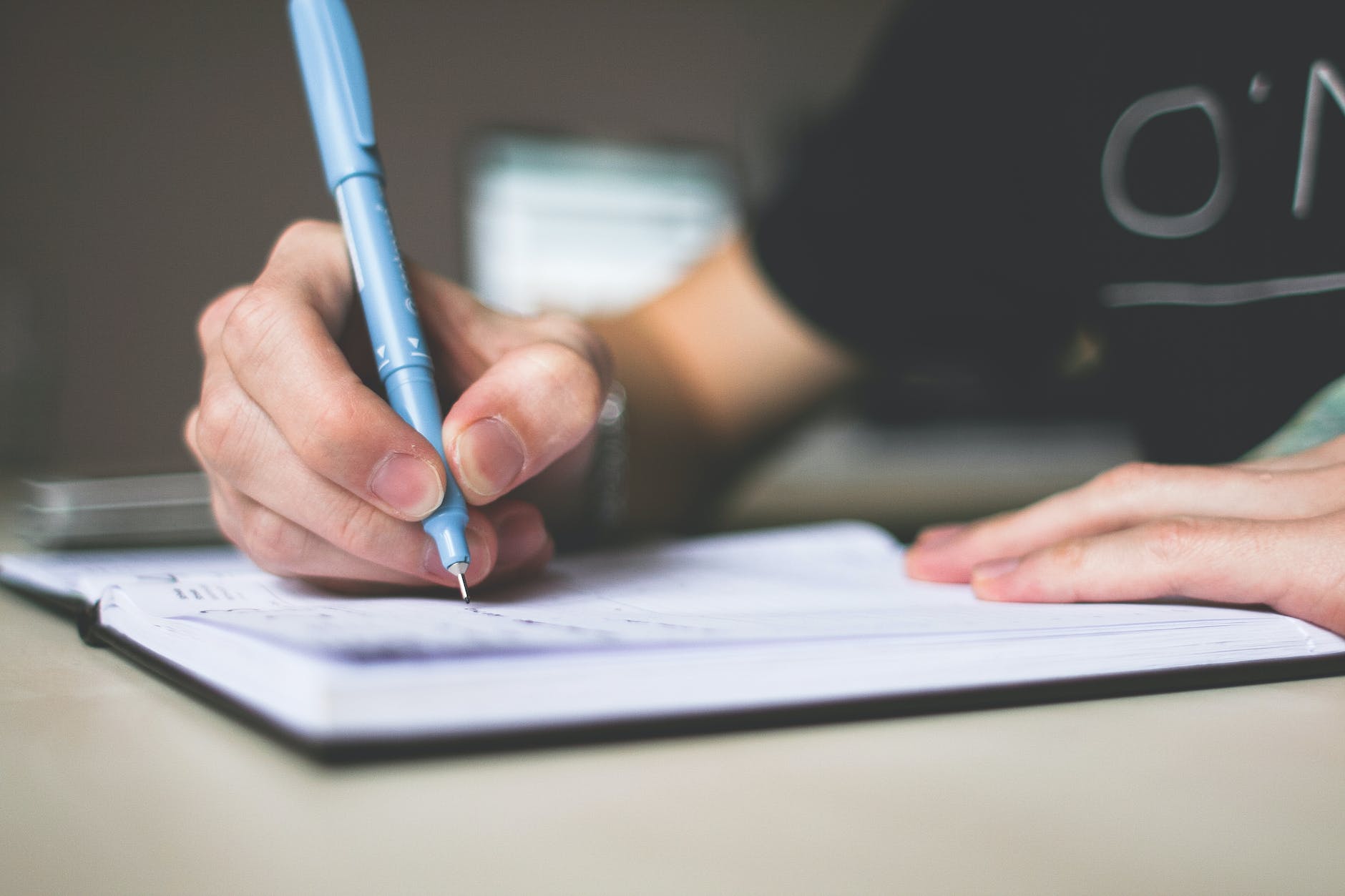
Safety Data Analytics: Leveraging Big Data for Proactive Risk Management
Introduction
In the ever-evolving landscape of safety engineering, the integration of safety data analytics has emerged as a powerful tool for proactive risk management. This article explores the significance of safety data analytics, its applications in mitigating risks, and how leveraging big data can revolutionize the way organizations approach safety in diverse industries.
Understanding Safety Data Analytics
Definition and Purpose
Safety data analytics involves the systematic analysis of large datasets related to safety incidents, near misses, and operational variables to extract meaningful insights. The primary purpose is to identify patterns, trends, and potential risk factors that may not be immediately apparent through traditional safety assessments.
Moving from Reactive to Proactive
Traditionally, safety management systems have been reactive, responding to incidents after they occur. Safety data analytics, on the other hand, enables a shift towards a proactive approach by identifying potential risks before they escalate into incidents.
Key Applications of Safety Data Analytics
Predictive Analytics for Incident Prevention
Predictive analytics uses historical safety data to forecast future incidents. By analyzing patterns and correlations, organizations can identify precursors to incidents and implement preventive measures, reducing the likelihood of accidents.
Real-Time Monitoring and Alerts
Safety data analytics allows for real-time monitoring of safety metrics. Automated alerts can be triggered based on predefined thresholds, enabling immediate intervention in situations where deviations from safety norms are detected.
Behavioral Analysis for Safety Culture Improvement
Analyzing safety data can provide insights into employee behaviors and adherence to safety protocols. This information is invaluable for shaping a positive safety culture by identifying areas for improvement and implementing targeted training programs.
Asset and Equipment Health Monitoring
Safety data analytics can extend to monitoring the health of assets and equipment. Predictive maintenance models can be developed to identify potential failures, minimizing downtime and preventing accidents caused by equipment malfunctions.
Leveraging Big Data in Safety Engineering
Integration of Diverse Data Sources
Big data in safety analytics involves integrating diverse data sources, including incident reports, environmental conditions, employee schedules, and equipment data. This comprehensive approach provides a holistic view of safety factors.
Machine Learning for Pattern Recognition
Machine learning algorithms can analyze vast datasets to recognize complex patterns and correlations. This capability is especially valuable in identifying subtle relationships that may contribute to safety risks.
Advanced Analytics for Root Cause Analysis
Big data analytics enables more sophisticated root cause analysis by considering a multitude of variables. It helps safety engineers go beyond immediate causes and delve into deeper, systemic issues contributing to incidents.
Challenges and Best Practices in Safety Data Analytics
Challenges
- Data Quality and Consistency: Ensuring the quality and consistency of safety data across diverse sources can be challenging.
- Data Privacy Concerns: Handling sensitive safety data requires careful consideration of privacy concerns and compliance with regulations.
- Integration of Legacy Systems: Integrating big data analytics with existing safety management systems may pose technical challenges.
Best Practices
- Data Standardization: Standardize data collection methods and formats to ensure consistency.
- Collaboration Across Departments: Foster collaboration between safety, IT, and data science departments to leverage the expertise of each.
- Continuous Improvement: Implement a culture of continuous improvement, using insights from analytics to enhance safety protocols.
Safety Data Analytics involves the use of advanced analytics techniques to analyze large volumes of data generated in the realm of safety and risk management. By leveraging Big Data, organizations can proactively identify patterns, trends, and potential risks, leading to more informed decision-making and enhanced safety measures. Here's a comprehensive overview of leveraging Safety Data Analytics for proactive risk management:
Key Components of Safety Data Analytics:
- Data Collection:
- Collect data from various sources, including incident reports, sensor data, employee feedback, and safety inspections.
- Ensure the data collected is diverse, covering different aspects of safety and risk.
- Data Integration:
- Integrate data from disparate sources into a unified platform or system.
- Use data integration tools to ensure data consistency and accuracy.
- Data Quality Assurance:
- Implement measures to ensure the quality and reliability of the data.
- Address data inconsistencies, errors, and missing information to maintain accuracy.
- Advanced Analytics Techniques:
- Utilize statistical analysis, machine learning algorithms, and predictive modeling to extract meaningful insights from the data.
- Apply data clustering, anomaly detection, and regression analysis to identify patterns and trends.
- Predictive Modeling:
- Develop predictive models to forecast potential safety incidents or identify areas of higher risk.
- Incorporate historical data to enhance the accuracy of predictions.
- Real-time Monitoring:
- Implement real-time monitoring systems that analyze data as it is generated.
- Enable timely detection of anomalies or deviations from normal safety conditions.
- Pattern Recognition:
- Identify patterns in safety data that may indicate specific risk factors.
- Use pattern recognition algorithms to uncover relationships between variables.
- Root Cause Analysis:
- Apply analytics to perform root cause analysis on incidents by tracing back to the underlying factors.
- Understand the systemic issues contributing to safety events.
Applications of Safety Data Analytics:
- Proactive Risk Identification:
- Anticipate potential safety risks by analyzing historical data and identifying early indicators.
- Implement preventive measures based on predictive analytics to mitigate risks.
- Behavioral Safety Analysis:
- Analyze employee behavior data to identify patterns related to safety compliance.
- Implement targeted training programs or interventions based on behavioral insights.
- Asset and Equipment Monitoring:
- Utilize sensor data and equipment monitoring to detect anomalies or signs of potential failures.
- Schedule proactive maintenance to address potential equipment-related safety risks.
- Incident Trend Analysis:
- Analyze historical incident data to identify trends and recurring patterns.
- Implement targeted interventions to address common issues and prevent future incidents.
- Workforce Safety Performance:
- Evaluate workforce safety performance by analyzing data related to near misses, training completion, and safety audits.
- Identify areas for improvement in safety culture and training programs.
- Emergency Response Optimization:
- Optimize emergency response plans by analyzing historical incident response data.
- Enhance resource allocation and response times based on predictive modeling.
- Compliance Monitoring:
- Monitor regulatory compliance by analyzing data related to safety inspections and audits.
- Implement corrective actions to address non-compliance issues.
- Safety Communication Effectiveness:
- Analyze the effectiveness of safety communication campaigns through employee feedback and incident response data.
- Adjust communication strategies based on analytics insights.
Benefits of Safety Data Analytics:
- Proactive Risk Management:
- Anticipate and address safety risks before they escalate into incidents.
- Efficient Resource Allocation:
- Optimize resource allocation based on identified risk areas and priorities.
- Continuous Improvement:
- Facilitate a culture of continuous improvement by learning from data insights.
- Cost Reduction:
- Minimize the financial impact of incidents by preventing them through proactive measures.
- Enhanced Decision-Making:
- Provide decision-makers with data-driven insights for more informed safety decisions.
- Increased Safety Awareness:
- Foster a heightened awareness of safety issues throughout the organization.
- Improved Compliance:
- Ensure compliance with safety regulations through proactive monitoring and adjustments.
Safety Data Analytics, when integrated effectively into an organization's safety management system, transforms data into actionable insights, ultimately contributing to a safer working environment and improved risk management.
Conclusion
In conclusion, safety data analytics, powered by big data, is transforming safety engineering into a proactive and data-driven discipline. By applying advanced analytics, machine learning, and real-time monitoring, organizations can gain unprecedented insights into safety risks, allowing for timely intervention and continuous improvement. Embracing safety data analytics is not just a technological shift; it’s a strategic move towards creating safer, more resilient workplaces.
Safety Data Sheets for Chemicals
What Is the Purpose of a Safety Data Sheet?
FAQs
- What is safety data analytics?
- Safety data analytics involves the systematic analysis of large datasets related to safety incidents, near misses, and operational variables to extract meaningful insights for proactive risk management.
- How does safety data analytics contribute to incident prevention?
- Predictive analytics in safety data analytics uses historical data to forecast future incidents, enabling organizations to identify potential risks and implement preventive measures.
- What role does big data play in safety engineering?
- Big data in safety engineering involves the integration of diverse data sources, machine learning for pattern recognition, and advanced analytics for root cause analysis, revolutionizing safety management systems.
- What challenges are associated with safety data analytics?
- Challenges include ensuring data quality and consistency, addressing data privacy concerns, and integrating big data analytics with existing legacy systems.
- What are some best practices for effective safety data analytics?
- Best practices include data standardization, collaboration across departments, and fostering a culture of continuous improvement using insights from analytics.